Translate this page into:
Sonographic Features of Chronic Kidney Disease in Agricultural Community in Sri Lanka
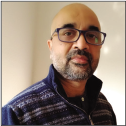
*Corresponding author: Janaka Wansapura, Advanced Imaging Research Center, University of Texas Southwestern, Texas, USA. janaka.wansapura@utsouthwestern.edu
-
Received: ,
Accepted: ,
How to cite this article: Bandara MS, Gurunayaka B, Lakraj GP, Pallewatte A, Siribaddana S, Wansapura J. Sonographic Features of Chronic Kidney Disease in Agricultural Community in Sri Lanka. Am J Sonogr 2021;4:1.
Abstract
Objectives:
The aim of this study was to use ultrasound-based kidney morphological features to classify chronic kidney disease (CKD) in an agricultural community in Sri Lanka where there is a high prevalence of CKD with unknown etiology.
Materials and Methods:
A cohort of CKD patients (n = 50) and healthy subjects (n = 26) underwent B-mode renal ultrasound. CKD patients were further categorized as those clinically diagnosed with diabetes mellitus, hypertension, and other known causes (n = 30) and those of unknown etiology (n = 20). Following kidney morphological features were calculated: Length (LEN), width (WDTH), cortical thickness, volume (VOL), and shape index.
Results:
CKD kidneys of both groups were significantly smaller than the healthy kidneys (P < 0.001). Based on a random forest procedure, the top three influential features that distinguished CKD kidneys from healthy kidneys were: VOL normalized to waist circumference (CKD = 0.6 ± 0.2 cm2, healthy = 0.9 ± 0.2 cm2), VOL normalized to body surface area (CKD = 36 ± 9 cm3/m2, healthy = 52 ± 13 cm3/m2), and WDTH (CKD = 3.6 ± 0.5 cm, healthy = 4.3 ± 0.6 cm). Patients with CKD of unknown etiology had higher kidney LEN and VOL normalized to height (HGHT) (LEN/HGHT = 0.58 ± 0.05 cm/m, VOL/HGHT = 0.40 ± 0.09 cm3/m, P < 0.05) compared to those of the known etiology group (LEN/HGHT = 0.51 ± 0.09 cm/m, VOL/HGHT = 0.30 ± 0.10 cm3/m).
Conclusion:
The study shows that ultrasound-based kidney volume can distinguish healthy versus diseased kidneys as well as CKD of known versus unknown etiology. Normalizing for height is required when comparing diseased groups.
Keywords
Chronic kidney disease
Kidney volume
Ultrasound
INTRODUCTION
Chronic kidney disease (CKD) is a global health concern with increasing prevalence in most countries.[1] Estimated glomerular filtration rate (eGFR), <60 ml/min/1.73 m2, increased albumin excretion, or both lasting for more than 3 months, is defined as CKD.[2] Hypertension, diabetes mellitus, and older age are among the classic risk factors of CKD.[3] However, in the past few decades, a new form of CKD known as either CKD of unknown etiology (CKDu)[4] or Chronic Interstitial Nephropathy among Agricultural Communities (CINAC)[5-7] has emerged among paddy farmers in the dry zone of Sri Lanka. CINAC is defined as a form of CKD that affects mainly young men, occasionally women.[6] The etiology of CINAC is thought to be mainly toxicological, occupational, and environmental.[8] Chemical compounds used in routine agricultural procedures such as pesticides and fertilizers including paraquat, glyphosate, and methamidophos were identified as the toxicological contributors in CINAC.[9,10] Further, the harsh agricultural employment conditions, drinking well water, family history of the disease, Ayurvedic treatments, and snake bites are considered as CINAC risk factors in Sri Lanka.[6,11] CKDu/CINAC does not present with commonly known risk factors for CKD such as diabetes, hypertension, glomerulopathies, or renal stone disease.[12] In 2013, Jayatilake et al. found the prevalence of CKDu/CINAC to be more than 15% in some districts of Sri Lanka.[12] CINAC is now found to be in several tropical countries including El Salvador, Nicaragua, Guatemala, and Costa Rica in Central America, Sri Lanka, and India in Asia, and Egypt in Africa.[5,6,13] The emergence of CINAC warrants a comparative study of the kidney morphological changes in both CKD with classic risk factors and CKD of unknown etiology (CINAC).
US imaging, considered the first-line diagnostic imaging procedure in CKD, provides several structural measurements of kidney, including length (LEN), width (WDTH), volume (VOL), and cortical thickness (THK).[14-18] Although it is widely accepted that the kidneys shrink in size as the disease progress and that the kidney size correlates with anthropometric parameters, there is no consent among investigators on the kidney features that should be used to represent the kidney size. According to Lucisano et al., parenchymal THK and kidney LEN normalized to body height (HGHT) show the highest correlation with kidney function in CKD.[19] However, in a larger population study of healthy kidneys, Emamian et al. found kidney VOL to be the most exact kidney measurement; with the highest correlation to the body surface area (BSA) (correlation coefficients = 0.595 and 0.600 for the left and right kidneys), whereas the normal kidney LEN correlated to the HGHT with correlation coefficients of 0.461 and 0.416 for the left and right kidneys.[18] In a study of healthy subjects and CKD patients, Jovanović et al. found that while kidney morphological features (e.g., WDTH, depth, VOL, etc.) correlated well with anthropometric parameters in healthy subjects, only VOL correlated significantly with the anthropometric parameters in CKD patients.[20]
Since existing studies do not consent to a single morphological feature and an appropriate correction for the body size, we analyzed feature vectors consisting of multitude of morphological features normalized to different anthropometric parameters such as BSA, body mass index (BMI), HGHT, and waist circumference (CIRC). Feature vectors were constructed from the most influential normalized features that discriminate given two groups, based on a random forest (RF) procedure.
RF classification
RF[21] is a classification and regression methodology based on the assembly of Classification and Regression Trees[22] which can be used as a supervised learning algorithm. RF fits many decorrelated trees on bootstrapped training sets and takes the most commonly occurring class among the fitted trees as the overall prediction in the classification context. In addition, RF uses mean decrease accuracy[21] as a metric to measure the importance of features in building a classifier. The importance of a feature is determined by how the accuracy decreases when the feature is excluded from the RF. Features with high mean decrease accuracy are the most influential predictors for the classifier.
MATERIALS AND METHODS
With the Institutional Ethics Review Committee approval, CKD patients (n = 79) undergoing routine US examination within a 6-month period at a regional hospital in Sri Lanka were randomly recruited for the study. All patients were clinically diagnosed with CKD according to the KDIGO criterion.[2] The eGFR of patients, calculated based on the serum creatinine level,[23] ranged from 14 ml/min/1.73 m2 to 87 ml/min/1.73 m2 with mean value of 49 ml/min/1.73 m2 at the time of the study. Patients with renal calculi, renal cysts, renal agenesis, and history of snake bites were excluded from the study. Total excluded patients were 29. Age matched healthy volunteers (n = 26) with no clinical symptoms and clinical history of kidney disease were recruited as a control group. Age, blood pressure, and anthropometric measurements (weight, HGHT, and waist CIRC) were collected from both CKD patents and healthy subjects before the US scan. Informed written consent was obtained before each examination from patients and healthy volunteers.
As per literature,[4,12] CKD patients diagnosed with hypertension, diabetes mellitus, or any other known cause were categorized as patients with known etiology (CKDKNOWN, n = 30) and the remaining kidney patients with no history of diabetes mellitus, chronic or severe hypertension, glomerular nephritis, or obstructive nephropathies were categorized as patients with unknown etiology (CKDUNKNOWN, n = 20).
Specialist radiologist performed US imaging. Toshiba Aplio 500 (Toshiba, Japan) US scanner with 3.5 MHz curved linear probe was used. Coronal and transverse views of the both left and right kidneys were taken without speckle reduction. Custom written MATLAB (The MathWorks, Inc., Natick, Massachusetts, United States) application was used to measure kidney features. Kidney measurements were manually performed under the supervision of an experienced radiologist. Measurements included; maximum longitudinal LEN measured in the coronal plane, maximum transverse WDTH measured in the coronal plane at the HGHT of the renal sinus, and the maximum THK measured in the transverse plane, as shown in Figure 1.

- A 60-year-old male patient with chronic kidney disease. (a) Renal ultrasound image indicates the maximum longitudinal length (white) and maximum transverse width (yellow) measured in the coronal plane, (b) maximum thickness (THK) measured in the transverse plane, (c) cortical THK measurements in the ultrasound image from the outer border of the kidney cortex to the outer border of the medulla.
Kidney VOL calculations were based on the ellipsoid formula,[24]
Volume = 0.5223×length×width (WDTH)×thickness (THK) (1)
Cortical THK was measured as the distance from the outer border of the kidney cortex to the outer border of the medullary pyramid. Three such measurements were taken as shown in Figure 1c and average cortical THK value was calculated. BMI[25] and the BSA[26] were calculated for all the subjects. Kidney shape alternations were determined by the ratio of kidney LEN to kidney WDTH. Measured kidney features; LEN, WDTH, and calculated kidney VOL were normalized to anthropometric parameters; body CIRC, HGHT, BMI, and BSA. Hereinafter, normalized features will be represented in the following format: Feature/ anthropometric parameter.
We used an RF procedure[21] to classify given two groups. To determine which features were more influential within the RF framework, the mean decrease accuracy (accuracy index) was calculated. The top (4–7) features in the accuracy index for a given classification problem were then used in a multivariate test for equality of mean vectors in the two group. Means of identified variables were compared using Hotelling’s T2 test and Bonferroni confidence intervals when the normality assumptions were accepted. When multivariate normality assumption was violated, bootstrap resampling method was used to compute P-values of the test and to obtain Bonferroni confidence intervals. Statistical analysis was performed using the R software package.[27]
RESULTS
Anthropometric details of the two groups (CKD n = 50, healthy subject n = 26) are listed in Table 1. The mean ± standard deviations of all morphological features in this study are given in Table 2.
CKD±SD (n=50) | Healthy subjects±SD (n=26) | |
---|---|---|
Male (%) | 56 | 54 |
Age (years) | 53±10 | 49±10 |
Weight, (kg) | 62±14 | 66±14 |
Height (cm) | 159±11 | 161±10 |
Waist circumference (cm) | 91±13 | 90±12 |
BMI (kg/m2) | 24±4 | 25±5 |
BSA (m2) | 1.6±0.2 | 1.6±0.2 |
CKD: Chronic kidney disease, BMI: Body mass index, BSA: Body surface area, SD: Standard deviation.
Feature | CKDKNOWN | CKDUNKNOWN | CKDall | Healthy |
---|---|---|---|---|
LEN (cm) | 8.2±0.2 | 9.2±0.9 | 8.7±0.1 | 10.0±0.8 |
WDTH (cm) | 3.5±0.4 | 3.7±0.4 | 3.6±0.5 | 4.3±0.6 |
VOL (cm3) | 53±16 | 64±15 | 58±17 | 87±25 |
ACT (cm) | 1.0±0.2 | 1.0±0.1 | 1.0±0.2 | 1.1±0.1 |
Shape index (LTW) | 0.44±0.06 | 0.4±0.1 | 0.42±0.06 | 0.43±0.05 |
LEN/CIRC | 0.9±0.2 | 1.0±0.2 | 0.9±0.2 | 1.1±0.1 |
LEN/BSA (cm/m2) | 52±8 | 57±6 | 54±8 | 61±7 |
LEN/BMI (cm m2/kg) | 3.5±0.7 | 3.8±0.8 | 3.6±0.7 | 4.0±0.7 |
LEN/HGHT (cm/m) | 0.51±0.09 | 0.58±0.05 | 0.55±0.09 | 0.62±0.06 |
WDTH/CIRC | 0.40±0.07 | 0.41±0.06 | 0.40±0.07 | 0.48±0.07 |
WDTH/BSA (cm/m2) | 22±3 | 23±3 | 23±3 | 26±3 |
WDTH/BMI (cm m2/kg) | 1.5±0.3 | 1.5±0.4 | 1.5±0.3 | 1.7±0.4 |
WDTH/HGHT (cm/m) | 0.22±0.03 | 0.23±0.02 | 0.22±0.03 | 0.27±0.03 |
VOL/CIRC (cm2) | 0.6±0.2 | 0.7±0.2 | 0.6±0.2 | 0.9±0.2 |
VOL/BSA (cm3/m2) | 33±9 | 39±8 | 36±9 | 52±13 |
VOL/BMI (cm3m2/kg) | 2.3±0.7 | 2.6±0.7 | 2.4±0.7 | 4±1 |
VOL/HGHT (cm3/m) | 0.3±0.1 | 0.40±0.09 | 0.4±0.1 | 0.5±0.1 |
CKD: Chronic kidney disease, LEN: Length, WDTH: Width, VOL: Volume, ACT: Average cortical thickness, LTW: Length to kidney width, LEN/BMI: Kidney length normalized to body mass index, LEN/BSA: Kidney length normalized to body surface area, LEN/CIRC: Kidney length normalized to body circumference, LEN/HGHT: Kidney length normalized to body height, VOL/BMI: Kidney volume normalized to body mass index, VOL/BSA: Kidney volume normalized to body surface area, VOL/CIRC: Kidney volume normalized to body circumference, VOL/HGHT: Kidney volume normalized to body height, WDTH/BMI: Kidney width normalized to body mass index, WDTH/BSA: Kidney width normalized to body surface area, WDTH/CIRC: Kidney width normalized to body circumference, WDTH/HGHT: Kidney width normalized to body height.
Healthy versus CKDALL
In this analysis, all CKD patients were grouped in to CKDALL. According to the accuracy index [Figure 2a], the top seven features that could distinguish CKDALL from healthy subjects in the descending order were VOL/CIRC, VOL/BSA, WDTH, VOL, VOL/BMI, LEN/CIRC, and WDTH/HGHT. Multivariate test for equality of mean vectors (permutation test) in the two groups showed a significant difference between healthy subjects and CKDALL patients (P < 0.001). According to 95% bootstrapped confidence intervals (Bonferroni corrected), taken independently, all seven features were significantly low in CKDALL compared to that of the healthy subjects (Table 2 for mean values). The 95% confidence interval of the mean difference between CKDALL and healthy of each feature is given in Table 3.

- Mean decrease accuracy of the random forest classifier for chronic kidney disease (CKD)ALL patients and healthy subjects (a) and CKDKNOWN and CKDUNKNOWN groups (b).
CKDALLpatients and healthy subjects | ||
---|---|---|
Lower limit | Upper limit | |
Feature | ||
VOL/CIRC | 0.194 | 0.465 |
VOL/BSA | 9.284 | 24.788 |
WDTH | 3.141 | 10.179 |
VOL | 16.069 | 46.062 |
VOL/BMI | 0.552 | 1.788 |
LEN/CIRC | 0.062 | 0.244 |
WDTH/HGHT | 0.021 | 0.061 |
CKDKNOWN patients and healthy subjects | ||
VOL/CIRC | 0.228 | 0.518 |
VOL/BSA | 10.940 | 27.400 |
WDTH | 3.045 | 10.837 |
VOL | 18.610 | 50.669 |
VOL/BMI | 0.654 | 1.955 |
LEN/CIRC | 0.092 | 0.297 |
WDTH/HGHT | 0.024 | 0.068 |
CKDUNKNOWNpatients and healthy subjects | ||
VOL/CIRC | 0.078 | 0.421 |
VOL/BSA | 3.354 | 22.406 |
WDTH | 1.458 | 10.443 |
VOL | 4.656 | 41.157 |
VOL/BMI | 0.136 | 1.699 |
LEN/CIRC | –0.034 | 0.207 |
WDTH/HGHT | 0.009 | 0.058 |
CKDKNOWN patients and CKDUNKNOWNpatients | ||
LEN/HGHT | 0.017 | 0.123 |
LEN/BSA | –0.147 | 10.105 |
VOL/BSA | –0.451 | 12.517 |
LEN/CIRC | –0.007 | 0.227 |
VOL/HGHT | 0.002 | 0.142 |
CKD: Chronic kidney disease, VOL: Volume, WDTH: Width, HGHT: Height, VOL/CIRC: Kidney volume normalized to body circumference, VOL/BSA: Kidney volume normalized to body surface area, WDTH: Kidney width, VOL: Kidney volume, VOL/BMI: Kidney volume normalized to body mass index, LEN/CIRC: Kidney length normalized to body circumference, WDTH/HGHT: Kidney width normalized to body height, LEN/HGHT: Kidney length normalized to body height, LEN/BSA: Kidney length normalized to body surface area, VOL/HGHT: Kidney volume normalized to body height.
Healthy versus CKDKNOWN
The same features as in the healthy versus CKDALL analysis were used to distinguish healthy subjects from CKDKNOWN patients. Multivariate test for equality of mean vectors (permutation test) in the two groups showed significant difference between healthy subjects and CKDKNOWN patients (P < 0.001). According to 95% bootstrapped confidence intervals (Bonferroni corrected), taken independently, all seven features were significantly low in CKDKNOWN patients compared to that of the healthy subjects. The 95% confidence intervals of the features are given in Table 3.
Healthy versus CKDUNKNOWN
The same seven features as in healthy versus CKDALL were used to distinguish healthy subjects from CKDUNKNOWN patients. Multivariate test for equality of mean vectors (Hotelling’s T2 test) in the two groups showed significant difference between healthy subjects and CKDUNKNOWN patients (P = 0.01). According to 95% Bonferroni confidence intervals, taken independently, except the kidney LEN normalized to CIRC (LEN/CIRC), other six features were significantly low in CKDUKNOWN patients compared to that of the healthy subjects. The 95% confidence intervals of the features are given in Table 3.
CKDKNOWN versus CKDUNKNOWN
RF classification of CKDKNOWN and CKDUNKNOWN groups found the highest accuracy index for LEN/HGHT, followed by LEN/BSA, VOL/BSA, LEN/CIRC, and VOL/HGHT [Figure 2b]. A multivariate test for equality of mean vectors in the two groups did not find a significant difference between CKDKNOWN patients and CKDUNKNOWN patients (P = 0.062). However, according to the 95% bootstrapped confidence intervals, taken independently, LEN normalized to HGHT and VOL normalized to HGHT were significantly different between the groups [Table 3]. On the other hand, the eGFR and age of CKDKNOWN (eGFR = 43 ± 20, age = 57 ± 3 years) patients were significantly different (p=0.01) to that of CKDUNKNOWN (eGFR = 57 ± 19, P = 0.02 age = 47 ± 7 years).
DISCUSSION
Our study demonstrates that US-based kidney morphological features in different combinations can distinguish healthy subjects and CKD patients. According to the RF model, kidney VOL normalized to waist CIRC, BSA, BMI, and kidney WDTH are the most important features in the classification. This is in line with the prior studies which report correlations between VOL and functional ability of kidney in different disease profiles.[28,29] The reduction in kidney VOL in CKD may be associated with known pathological alternations such as glomerular sclerosis and tubular atrophy.[30]
Serum creatinine level of healthy subjects was not measured in this study, which could be considered a limitation, as their kidney health could not be verified biochemically.
Our results show that the normal kidney LEN (10 ± 1 cm) of Sri Lankan population is similar to that of other Asian countries; Northwest Indians = 10.0 cm and Pakistanis = 10.4 cm. The kidney VOL (87 cm3) of Sri Lankans as reported in this study appears to be smaller than that of Danish (140 cm3), Korean (158 cm3), and Caucasian (130 cm3) ethnicities.[31]
In this study, we identified two groups of CKD patients on their clinical presentation at the time of the study. One group of patients presented with classical risk factors (CKDKNOWN), and the etiology of the other CKD group was unknown (CKDUNKNOWN). The etiology of the CKDUNKNOWN group cannot be determined from the available data but could be hypothesized based on the literature as CINAC (or CKDu) patients. The present study population was drawn from a region of high prevalence of CINAC[10] and CKDUNKNOWN patients did not have any of the classical risk factors. Therefore, it is very likely that CKDUNKNOWN patients may be clinically defined as CINAC patients. However, it was not able to verify this retrospectively and can be regarded as a limitation of this study. It should also be pointed out that since all CKD patients in this study were from CINAC endemic areas, some of the CKDKNOWN patients could have complex etiologies.
We found that the kidney LEN normalized to HGHT and VOL normalized to HGHT were significantly different between CKDUNKNOWN and CKDKNOWN. However, CKDUNKNOWN patients were younger, had higher eGFR, and did not have any of the classical risk factors.
CONCLUSION
It is likely that the progression and the severity of CKDUNKNOWN (possibly CINAC) are different to that of the CKDKNOWN.
Ultrasound-based kidney morphological measurements in combination with RF classification could play a key role in future studies of disease progression in this group.
Declaration of patient consent
The authors certify that they have obtained all appropriate patient consent.
Financial support and sponsorship
National Research Council, 120/07, Vidya Mawatha, Colombo 07, Sri Lanka (Grant Number: NRC 16-044).
Conflicts of interest
There are no conflicts of interest.
References
- Chronic kidney disease: Global dimension and perspectives. Lancet. 2013;382:260-72.
- [CrossRef] [Google Scholar]
- KDIGO 2012 clinical practice guideline for the evaluation and management of chronic kidney disease. Kidney Int Suppl. 2013;3:27.
- [Google Scholar]
- Association of fibroblast growth factor 23 with atrial fibrillation in chronic kidney disease, from the chronic renal insufficiency cohort study. JAMA Cardiol. 2016;5:548-56.
- [CrossRef] [PubMed] [Google Scholar]
- Arterial stiffness and Sri Lankan chronic kidney disease of unknown origin. Sci Rep. 2016;6:32599.
- [CrossRef] [PubMed] [Google Scholar]
- Global dimensions of chronic kidney disease of unknown etiology (CKDu): A modern era environmental and/or occupational nephropathy? BMC Nephrol. 2015;16:145.
- [CrossRef] [PubMed] [Google Scholar]
- Chronic interstitial nephritis in agricultural communities: A worldwide epidemic with social, occupational and environmental determinants. Nephrol Dial Transplant. 2017;32:234-41.
- [CrossRef] [PubMed] [Google Scholar]
- Chronic interstitial nephritis in agricultural communities is a toxin-induced proximal tubular nephropathy. Kidney Int. 2020;97:350-69.
- [CrossRef] [PubMed] [Google Scholar]
- Toward a comprehensive hypothesis of chronic interstitial nephritis in agricultural communities. Adv Chronic Kidney Dis. 2017;24:101-6.
- [CrossRef] [PubMed] [Google Scholar]
- Importance of arsenic and pesticides in epidemic chronic kidney disease in Sri Lanka. BMC Nephrol. 2014;15:124.
- [CrossRef] [PubMed] [Google Scholar]
- Glyphosate, hard water and nephrotoxic metals: Are they the culprits behind the epidemic of chronic kidney disease of unknown etiology in Sri Lanka? Int J Environ Res Public Health. 2014;11:2125-47.
- [CrossRef] [PubMed] [Google Scholar]
- Chronic renal failure in North Central Province of Sri Lanka: An environmentally induced disease. Trans R Soc Trop Med Hyg. 2007;101:1013-7.
- [CrossRef] [PubMed] [Google Scholar]
- Chronic kidney disease of uncertain aetiology: Prevalence and causative factors in a developing country. BMC Nephrol. 2013;14:180.
- [CrossRef] [PubMed] [Google Scholar]
- Chronic interstitial nephritis in agricultural communities (CINAC) in Sri Lanka. Semin Nephrol. 2019;39:278-83.
- [CrossRef] [PubMed] [Google Scholar]
- The role of ultrasonography in the study of medical nephropathy. J Ultrasound. 2007;10:161-7.
- [CrossRef] [PubMed] [Google Scholar]
- Renal relevant radiology: Use of ultrasound in kidney disease and nephrology procedures. Clin J Am Soc Nephrol. 2014;9:373-81.
- [CrossRef] [PubMed] [Google Scholar]
- Ultrasound Doppler renal resistive index: A useful tool for the management of the hypertensive patient. J Hypertens. 2014;32:149-53.
- [CrossRef] [PubMed] [Google Scholar]
- Renal ultrasound elastography. Diagn Interv Imaging. 2013;94:545-50.
- [CrossRef] [PubMed] [Google Scholar]
- Intraobserver and interobserver variations in sonographic measurements of kidney size in adult volunteers. A comparison of linear measurements and volumetric estimates. Acta Radiol. 1995;36:399-401.
- [CrossRef] [PubMed] [Google Scholar]
- Can renal sonography be a reliable diagnostic tool in the assessment of chronic kidney disease? J Ultrasound Med. 2015;34:299-306.
- [CrossRef] [PubMed] [Google Scholar]
- Correlation of kidney size with kidney function and anthropometric parameters in healthy subjects and patients with chronic kidney diseases. Ren Fail. 2013;35:896-900.
- [CrossRef] [PubMed] [Google Scholar]
- Classification and regression trees In: Classification and Regression Trees. New York: CRC Press; 1984. p. :368.
- [Google Scholar]
- A more accurate method to estimate glomerular filtration rate from serum creatinine: A new prediction equation andrew. Ann Intern Med. 2009;130:241-52.
- [CrossRef] [PubMed] [Google Scholar]
- Sonographic measurements and appearance of normal kidneys in children. AJR Am J Roentgenol. 1985;145:611-6.
- [CrossRef] [PubMed] [Google Scholar]
- Relationships between preptin and osteocalcin in obese, overweight, and normal weight adults. Appl Physiol Nutr Metab. 2015;40:218-22.
- [CrossRef] [PubMed] [Google Scholar]
- Body surface area: Du bois and du bois revisited. Eur J Appl Physiol. 2000;82:250-4.
- [CrossRef] [PubMed] [Google Scholar]
- Preoperative renal volume: A surrogate measure for radical nephrectomy-induced chronic kidney disease. J Endourol. 2015;29:1406-11.
- [CrossRef] [PubMed] [Google Scholar]
- Total kidney volume as a biomarker of disease progression in autosomal dominant polycystic kidney disease. Can J Kidney Heal Dis. 2017;4:1-6.
- [CrossRef] [PubMed] [Google Scholar]
- Tubular atrophy in the pathogenesis of chronic kidney disease progression. Pediatr Nephrol. 2016;31:693-706.
- [CrossRef] [PubMed] [Google Scholar]
- Comparison of renal size among different ethnicities. Int J Biol Biomed Eng. 2011;5:221-9.
- [Google Scholar]